1. Introduction
In recent decades, climate change and socio-economic growth have had impacts on available water resources and food security in most parts of the world (Biemans et al. 2013; Lee, Bae 2015). Thus, more sophisticated management of existing water resources (Mereu et al. 2016; Wałęga et al. 2020), environments (Alam et al. 2018), and ecosystems (Yaegashi et al. 2018) is required to adapt to the impacts. A water reservoir’s operation should follow a rational policy to ensure adequate water provision for its various intended purposes without adverse effects. Figure 1 shows a conceptual diagram of a typical reservoir operation system in the context of feedback control theory. Hydrologic inputs, including precipitation, evapotranspiration, and runoff from the catchment, affect the reservoir’s water balance and the water supply to the command area (i.e. the downstream area serviced by the reservoir’s functions). The release from the reservoir as a control variable also contributes to the reservoir’s water balance and the water supply to the command area. The operator’s role is to implement a policy determining the release discharges based on the reservoir’s water level and the water demand from the command area. However, a more sophisticated system of reservoir operation might also account for hydrologic inputs and/or possible conveyance losses as parts of the information on which the policy is based.
Labadie (2004), Rani and Moreira (2010), and Ahmad et al. (2014) reviewed a variety of methods for computationally determining suitable reservoir operation policies. Stochastic dynamic programming (SDP) theory is the general framework to establish reservoir operation policies, as explored decades ago (Heidari et al. 1971; Tejada-Guibert et al. 1993, 1995; Yakowitz 1982). The SDP typically provides optimal operation policies with thresholds of opening valves or switching pumps (Nop et al. 2021; Unami, Mohawesh 2018; Unami et al. 2019b). However, reservoir operators generally do not accept such theoretically generated policies but instead rely on their empirical knowledge to make decisions when unexpected risks are involved (El-Shafie et al. 2014). Therefore, attention should be paid to the actual policies that may be identified from historical data (Giuliani et al. 2014). Turner et al. (2021) firstly attempted to inventory reservoir operation policies in the United States.
This study aims at establishing a new approach to identifying nominal release policies implemented in a multi-purpose water reservoir. Bukit Merah Reservoir (BMR), located in the northern part of Perak State, Malaysia, was chosen as a study site. The water levels and release discharges of BMR were recorded daily from 2000 through 2011. Generalized additive models (GAMs; Chen, Tsay 1993) are applied for representing the operational policies for release discharges, which are assumed to have an annual period based on the day of the year and the water levels (Unami et al. 2019a). The backfitting algorithm works well to identify each contributing function of the GAMs (Breiman, Friedman 1985). However, the release policies identified with that method include spurious oscillations, which are removed with total variation regularization (TVR), known as the Rudin–Osher–Fatemi model (ROF) for the initials of its three authors (Rudin et al. 1992). The ROF model contains a scale parameter dominating the discrepancy of the reconstructed data from the original data that includes spurious oscillations (Osher et al. 2005). Further application of TVR in agricultural water management was discussed in Fadhil and Unami (2021). The identified release policies, which are considered nominal because they have been smoothed and regularized, are utilized to examine shifts in the operation of BMR during the period. Several factors stemming from climate change and socio-economic growth are inferred to have burdened the operation of BMR with more demanding release policies.
2. Materials and methods
2.1. Study site and datasets
Figure 2 shows the topography of BMR’s catchment and command areas, extracted from the Shuttle Radar Topography Mission (SRTM) digital elevation data (Farr et al. 2007), with the boundaries of sub-catchments demarcated with white lines. The climatic zone is tropical rainforest with a mean annual rainfall of more than 3000 mm. The bimodal annual rainfall pattern enables paddy rice cultivation twice a year. BMR has a catchment area of 480 km2; it supplies irrigation water to the paddy fields of the Kerian Irrigation Scheme (KIS), covering an area of 236 km2, and urban water for municipal and industrial purposes to the Kerian, Larut, Matang, and Selama Districts. The catchment includes four river systems: Merah, Jelutong, Selarong, and Kurau Rivers (Ismail, Najib 2011), located between 04 51 N and 05 10 N latitude and 100 38 E to 101 00 E longitude. The region is primarily rural with numerous riverine villages established along the middle and lower reaches of the rivers. The land use in the areas with elevations lower than 50 m is predominantly for tree plantations such as oil palm, rubber, and coconut. At the same time, the surrounding steep mountain slopes are covered with rainforests. The rainfall characteristics in the catchment area have been modeled with a stochastic generator (Fadhil et al. 2017). Analysis using rainfall-runoff models with fractional derivatives has shown that the hydrologic response in the catchment area of the Kurau River is unstable (Unami et al. 2021). The KIS’s 236 km2 command area consists of low-lying lands between the Strait of Malacca and BMR. Two primary irrigation canals, the Main Canal and the Selinsing Canal, convey the irrigation water by gravity. The urban water is taken from the Selinsing Canal at a pumping station located 6.5 km downstream of BMR. Dor et al. (2011) conducted a detailed hydro-geological study on the Selinsing Canal. Approximately 61% of water consumed in the paddy fields of the KIS originates from BMR, and the rest is from rainfall (Hamidon et al. 2015). Urban demand depends on the local population and its economy. The Department of Irrigation and Drainage (DID, 2011) projected that the growth rates of the population and economy in the state of Perak would decrease but remain positive until the year 2050. Intensive research on the operation of BMR has been conducted in the context of future climate change and SDP (Fadhil 2018). Besides the irrigation and urban demands, the operation of BMR needs to account for flood control and environmental hazards. There are two gated spillways of Ogee weir type with the same crest level, draining water from BMR to the original water course of the Kurau River, which meanders through the KIS with widths of 30-50 m.
The key dimensional parameters of BMR are summarized in Table 1. The dam embankment is at an elevation above the sea level (EL) of 11.28 m. The datum level is taken as the lowest level of the reservoir. The water level above the sea level is denoted by h (m). The storage volume of the reservoir when the water level is equal to h is denoted by V(h). The maximum capacity of the spillways at MWL is 565 m3/s. However, the bed slope of the Kurau River receiving the water from the spillways is about 1/10,000, implying insufficient discharge capacity.
Table 1
Key dimensional parameters of BMR.
A daily log of the operation of BMR in terms of water levels, rainfall depths, and release discharges into the two primary irrigation canals for the period from 2000 through 2011 was provided from the DID. The complete source data are available in a supporting information file.
Hamidon et al. (2015) estimated the irrigation demand Qirr in the KIS for each month. The urban demand Qurb was assumed to be constant at 1.70 m3/s (Anwar 2010). Then, the sum of the irrigation demand Qirr and the constant urban demand Qurb becomes the total demand discharge QD for each month. Table 2 summarizes the average monthly rainfall depths R during 2000-2011, the values of Qirr, Qurb, and QD, and the observed average monthly release discharge QR. However, it is noteworthy that the actual irrigation demand varies on a daily scale, according to the GIS-based estimation by Rowshon et al. (2003a-b).
Table 2
Monthly parameter values relevant to the water balance of BMR.
2.2. Generalized additive models for release discharges
The water level of BMR at the beginning of day t is denoted by ht. The release discharge into the primary irrigation canal k on the day t of the year y is denoted by
where
the function
in the sense of the least squares as well as the TV.
2.3. Backfitting algorithm
Firstly, the backfitting algorithm is applied for finding the functions to minimize the expected square error
and
for integers l with the increments Δt and Δh, respectively. Then, with initial guesses
and
where Ck are taken as the averages of all
2.4. TVR
After the backfitting algorithm has converged, the TVR is applied to each
where λ is the scale parameter of the ROF model, and ∇ = ∂/∂x. The Euler-Lagrange equation for minimization of J in (8) is formally written as:
We attempt to obtain an approximate solution to (9) by numerically solving the initial value problem of the singular diffusion equation:
It is assumed that the values of f are determined at n different points of Ω. Let those points be xi for i = 0, 1, …, n – 1 such that x0 < x1 < … < xn–1. Applying the standard Galerkin finite element scheme with piecewise linear bases to (10) results in the initial value problem of the ordinary differential equation:
where u ∈ ℝn is the vector whose ith entry is
(12)
and
For the Neumann boundary condition, the matrices become:
(14)
and
3. Results
3.1. Identification of nominal release policies and reconstruction of release discharges
Two five-year subperiods, 2001-2005 and 2007-2011, were extracted from the whole period 2000-2011. Consecutive use of the backfitting algorithm and the TVR identified nominal release policies from the observed release discharges during each of the two subperiods. The increments are chosen as
Fig. 3.
The process of TVR applied to the function of time for the Main Canal during the subperiod 2001-2005.
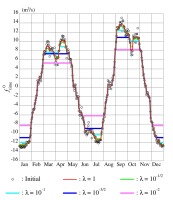
Fig. 4.
The process of TVR applied to the function of time for the Main Canal during the subperiod 2007-2011.
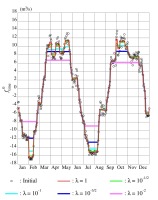
Fig. 5.
The process of TVR applied to the function of WL for the Main Canal during the subperiod 2001-2005.
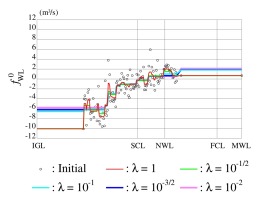
Fig. 6.
The process of TVR applied to the function of WL for the Main Canal during the subperiod 2007-2011.
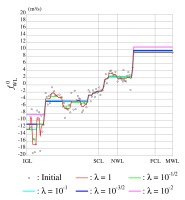
Fig. 9.
The process of TVR applied to the function of time for the Selinsing Canal during the subperiod 2001-2005.
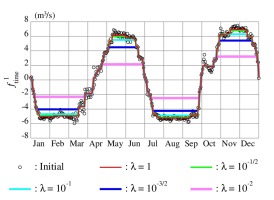
Fig. 10.
The process of TVR applied to the function of time for the Selinsing Canal during the subperiod 2007-2011.
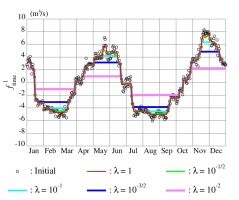
Fig. 11.
The process of TVR applied to the function of WL for the Selinsing Canal during the subperiod 2001-2005.
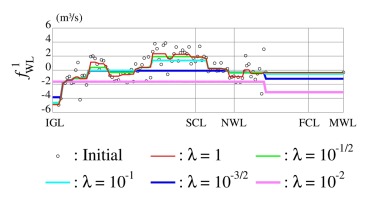
3.2. Choice of the scale parameter
The daily oscillations in
4. Discussions
4.1. Comparison of the two subperiods
The comparison between the observed and the reconstructed release discharges during the whole period validates the release policies identified from the data during each of the two subperiods. Significant increases in the peaks and the TV of the observed release discharges in both canals can be seen in 2009-2011. Consequently, the reconstructed release discharges with the 2001-2005 policies barely approximate the observed ones in 2009-2011. This indicates that the operator changed the release policies from the subperiod 2001-2005 to the subperiod 2007-2011.
Referring again to Figure 1, the variable hydrological input, which is under the considerable influence of climate change, is considered the primary cause of changes in the release policies controlling the water balance in the reservoir to satisfy the water demand.
The changes in the functions
The function
4.2. Comparison with other approaches
The first key feature of the approach developed here is to decompose a release policy into possibly singular functions of a single independent variable with the additive structure, unlike the standard procedure identifying release policies as quite regular functions of multiple independent variables (Turner et al. 2021). As discussed in the previous subsection, the decomposition of release policies illustrates the two aspects of the irrigation demand’s annual patterns and the hydraulic structures’ functions.
The second key feature is the TVR applied to the decomposed possibly singular functions, breaking through a widespread obsession that policy identification is “to minimize some distance metric between historical releases and modeled ones,” as in Giuliani et al. (2014). This study is the first to succeed in quantitatively treating the indecisive attitude of a reservoir operator as the spurious oscillations to be removed with the TVR. The release policies extracted with the TVRs are the nominal parts. Overgaard (2019) provides mathematical proof to endorse the ROF model in one dimension used in this study for TVR. However, the lack of an established method to choose the best scale parameter λ is a practical limitation of this approach. A follow-up study shall be conducted to validate the λ values with the empirical knowledge of the reservoir operator.
A sophisticated approach is being developed to combine the backfitting algorithm with TVR (Yang, Tan 2018). Nevertheless, our approach, opting for the sequential use of the two procedures, is advantageous because it is not computationally demanding.
5. Conclusions
The consecutive use of the backfitting algorithm and TVR successfully identified the nominal release policies implemented in BMR during each of the two subperiods as the GAMs. The additive structure decomposed the varying parts of the nominal release policies into the two functions. The scale parameter of the TVR controlling the removal of spurious oscillations should be chosen so that the nominal release policies are sensitive to the time and water levels. The results have been utilized to compare the two subperiods, indicating the significant changes in the operator’s release policies. The rapidly developing agronomic practices and the insufficient discharge capacity of the Kurau River receiving the water released from the spillways might be seriously burdening the operation of BMR. We have also addressed the situation in another study (Fadhil 2018), so that the reservoir operator is aware of future hydro-meteorological information, and deducing optimal release policies of BMR based on the SDP theory.